What is Retrieval-Augmented Generation (RAG) and How It Enhances AI Capabilities
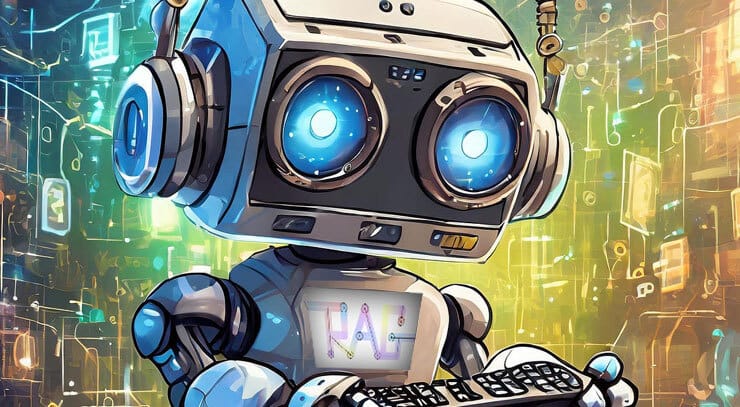
Have you ever wondered how artificial intelligence (AI) keeps getting smarter? It’s not just magic, there's a whole lot of technology working behind the scenes. One such technology is Retrieval-Augmented Generation (RAG). In this blog, you'll get a solid understanding of what RAG is and how it’s transforming AI. RAG is like a brainy assistant that doesn’t just rely on its memory but also knows where to find the best information in real time. Let’s get into this and see how RAG is enhancing its capabilities in ways we never thought possible.
What is Retrieval-Augmented Generation (RAG)?
Retrieval-Augmented Generation (RAG) is an advanced AI technology that combines retrieval and generation processes to provide accurate and contextually relevant information. Retrieval involves searching through the databases to find the most relevant data, while generation creates new content based on the AI's existing knowledge.
With the integration of these two functions, RAG allows AI to fetch the best information from large datasets and generate precise responses. This combination enhances the AI's ability to deliver up-to-date and comprehensive answers, making it a powerful tool in various applications like research, customer support, and more.
How Does RAG Work?
Now, let’s understand how RAG works. Imagine you ask an AI a question about quantum physics. Instead of relying only on pre-learned knowledge, the AI uses RAG to enhance its response. Here’s a step-by-step breakdown of what happens:
1. Query Understanding: The AI begins by comprehending your question, utilizing natural language processing (NLP) to understand the subtleties and specifics of your query, which is crucial for retrieving the correct information.
2. Retrieval Phase: Once the question is understood, the AI searches through a vast database or external sources to find the most relevant pieces of information.
3. Generation Phase: After gathering the relevant information, the AI then generates a response. This isn’t just a cut and paste job, the AI synthesizes the information to create a contextually appropriate answer.
4. Response Delivery: Finally, the AI delivers the answer to you in a clear and understandable manner. The result is a response that’s not only informative but also accurate and detailed.
How Can RAG Enhance AI Capabilities?
Now let’s see how Retrieval-Augmented Generation (RAG) is transforming AI and enhancing its capabilities. We’ll explore its benefits making it easy to understand the significant impact of this technology.
1. Enhanced Accuracy and Relevance
One of the primary benefits of RAG is its ability to provide more accurate and relevant information. Traditional AI models rely solely on the data they were trained on, which can become outdated quickly. In contrast, RAG can access up-to-date information from vast external databases, ensuring the responses it generates are both current and precise. This is especially important in dynamic fields such as medicine, finance, and technology, where new information is continually emerging. By retrieving the latest data and generating responses based on it, RAG significantly enhances the accuracy and relevance of AI outputs.
2. Broader Knowledge Base
Traditional AI models are limited by the size and scope of their training data. Once trained, they cannot access new information unless they are retrained with updated datasets. RAG overcomes this limitation by incorporating a retrieval mechanism that can tap into expansive external databases in real-time. This means RAG-powered AI systems have a virtually limitless knowledge base, allowing them to handle a wider range of topics with greater depth and detail. Whether it’s a niche academic query or a complex technical question, RAG can provide informed answers by leveraging its extensive retrieval capabilities.
3. Improved Context Understanding
Context is important when generating meaningful and coherent responses. Traditional AI models often struggle with understanding and maintaining context, especially in long and complex interactions. RAG, however, excels in this area by retrieving contextually relevant information before generating a response. This ensures that the generated content is not only accurate but also contextually appropriate. For instance, in a customer support scenario, RAG can retrieve relevant case histories and knowledge articles, ensuring that the response is tailored to the specific context of the customer’s query. This level of contextual understanding greatly enhances the quality of AI interactions.
4. Scalability and Adaptability
As new information becomes available, traditional AI models require extensive retraining to incorporate this data. This process is time-consuming and resource-intensive. RAG, on the other hand, is scalable and adaptable. Its retrieval component can instantly access and incorporate new information from external sources without the need for retraining. This makes RAG-powered AI systems highly adaptable to changing information landscapes. In fields where information evolves rapidly, such as scientific research or market trends, RAG ensures that AI remains current and useful, providing reliable and up-to-date insights.
5. Enhanced Decision-Making
By combining retrieval and generation, RAG empowers AI systems to make better-informed decisions. In scenarios where decision-making depends on the latest data and nuanced understanding, RAG can retrieve the necessary information and generate insightful, informed recommendations. For example, in healthcare, RAG can assist doctors by retrieving the latest research and treatment protocols and generating tailored recommendations for patient care. This capability extends to various other domains, including finance, law, and business, where informed decision-making is important.
6. Versatility in Applications
The versatility of RAG is another significant advantage. It can be applied across a wide range of industries and use cases, from customer support and academic research to content creation and legal advice. In customer support, RAG can provide accurate and contextually relevant responses to customer queries, enhancing the overall support experience. In academic research, RAG can assist researchers by retrieving and synthesizing information from vast scientific databases, enabling more thorough and informed research. Content creators can leverage RAG to generate high-quality, well-researched content by pulling in relevant information from various sources. The potential applications of RAG are vast and varied, making it a valuable tool in numerous fields.
Conclusion
Retrieval-Augmented Generation is a powerful tool that’s enhancing the capabilities of AI in remarkable ways. By combining the strengths of retrieval and generation, RAG allows AI systems to provide more accurate, contextually relevant, and up-to-date responses. Whether it’s in healthcare, customer support, legal research, or content creation, the applications of RAG are vast and varied.
As we move forward, the integration of RAG with other AI technologies promises to unlock even greater potential, creating the way for a future where AI can truly understand and assist us in ways we never thought possible. So, the next time you interact with an AI, remember that behind the scenes, technologies like RAG are working hard to bring you the best possible answers!
At Meii AI, we leverage this very technology to power our advanced conversational AI models. Our platform integrates RAG to deliver precise and context-aware responses. By combining world-class large language models with innovative retrieval techniques, Meii AI sets a new standard in AI-driven conversations. This ensures that our clients receive the most accurate and insightful interactions, driving true value and providing greater insights.